By Ian David Moss on February 5th, 2014
Fractured Atlas as a Learning Organization: You’re Not as Smart as You Think
(This is the second post in a series on our capacity-building pilot initiative, Fractured Atlas as a Learning Organization. To read more about it, please check out Fractured Atlas as a Learning Organization: An Introduction.)
Last fall, we put together a group of six people (henceforth referred to as the Data-Driven D.O.G. Force) to collectively develop a set of decision-making frameworks to help us resolve so-called decisions of consequence — situations for which the level of uncertainty and the cost of being wrong are both high. To do this, we’ve been taking inspiration from Doug Hubbard’s book How to Measure Anything, which introduces a concept he invented called “applied information economics,” or AIE. AIE is a formalized method of building a quantitative model around a decision and analyzing how information can play a role in making that decision. You can read much more about it in Luke Muehlhauser’s excellent summary of How to Measure Anything for Less Wrong.
One of the central tenets of AIE is that we can only judge the value of a measurement in relation to how much it reduces our uncertainty about something that matters. (More on that in a future post!) In order to know that, though, we have to have some sense of how much uncertainty we have now. This concept of uncertainty is one that we understand on an intuitive level — I might be much more confident, say, predicting that I’ll be hungry at dinner-time tonight than predicting what I’ll be doing with my life 10 years from now.
But most people don’t have a lot of experience quantifying their uncertainty. And yet, as forecasting experts from Hubbard to Nate Silver tell us, the secret to successful predictions (or at least less terrible predictions) is thinking probabilistically. What does this mean in practice? Picture yourself at Tuesday trivia night at your favorite local pub. There you are with your teammates, you’ve come up with some ridiculous name for yourselves (like, I don’t know, the “Data-Driven D.O.G. Force”), and the round is about to begin. The emcee calls out the question: “the actor Tom Cruise had his breakout role in what 1983 movie?” Your friend leans over and says, “It’s Risky Business. I’m like 99% sure.” Anyone who’s done time at trivia night will probably recognize something like that sequence.
What I can virtually guarantee you, though, is that your friend in this situation hasn’t thought very hard about that 99% figure. Is it really 99%? That’s awfully confident — it implies that if your friend were to answer 100 questions and was as confident about every one of the answers as she was about this one, she would be right 99 times. I’d be willing to bet that if you recorded the number of times people said they were “99% sure” about something and kept track of how often they were actually right, it would be significantly less than 99% of the time.
That’s because as human beings, we tend to be overconfident in our knowledge in all sorts of ways, and this exact effect has been documented by psychologists and behavioral economists in experiment after experiment for decades. This is why any AIE process involves something called calibration training. Overconfidence is an endemic and hard-to-escape problem, but if you practice making predictions and confront yourself with feedback about the results of those predictions, you can get better. In How to Measure Anything, Hubbard provides a number of calibration tests essentially consisting of trivia questions like the one above — except that instead of naming a specific movie or person, we’re asked to provide a ranged estimate (for numbers) or a confidence rating in the truth or falsehood of a statement. So for example, you might find yourself guessing what year Risky Business came out, or whether it’s true or false that it was Tom Cruise’s first leading role.
The six of us on the D.O.G. Force took a number of these calibration tests, and I’m gonna be honest with you — we were pretty awful. We got the hang of the binary (true/false) predictions relatively quickly, but the ranged estimates proved exceedingly difficult for us. In four iterations of the latter test across six individuals, only one of us ever managed to be right more often than we said we would be. You can see this in the results below (red colors and negative numbers mean that we were overconfident, green colors and positive numbers underconfident, and yellow/zero right on the money).
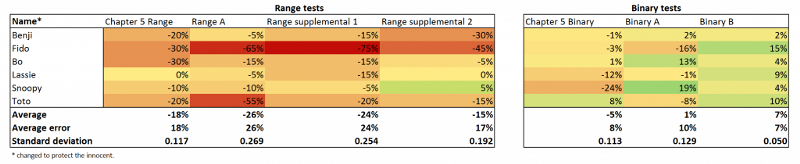
We were able to make good progress in the last round of the test (“Range supplemental 2”), though, primarily by focusing on making our ranges wide enough when we really had no idea what the right answer was. What’s the maximum range of a Minuteman missile? Well, if you don’t even know what a Minuteman missile is, your range should be wide enough to cover everything from a kid’s toy to an ICBM. It can feel incredibly unsatisfying to admit that the range of possibilities is so wide, but in order to construct an accurate model of the state of your knowledge, right now, you need to be able to articulate what “I have no idea” really means.
So why spend valuable company time working through a bunch of trivia questions? Because when we find ourselves needing to make estimates about, say, how much a new software feature might cost, or the number of people who might be reached when we speak at a conference, we suffer from the same disease of overconfidence if we don’t do something about it. What happens as a result is that we make predictions that are reassuringly precise in the moment, but might well end up far off from reality down the road. And when we use those inaccurate assumptions and predictions in our decision-making, there’s a good chance — so to speak — that we’re setting ourselves up for later regret. Next up: how this all fits in with grand strategy! [UPDATE: If you want to try a range test for yourself, our rockstar Community Engagement Specialist and D.O.G. Force member Jason Tseng has created an arts-specific one! Here are the questions and here are the answers (don’t peek!).]
About Ian David Moss
Ian David Moss was the Senior Director of Information Strategy at Fractured Atlas, a nonprofit technology company that helps artists with the business aspects of their work. To learn more about Fractured Atlas, or to get involved, visit us here.